Functional Data Analysis with R Chapman & Hall/CRC Monographs on Statistics and Applied Probability Series
Auteurs : Crainiceanu Ciprian M., Goldsmith Jeff, Leroux Andrew, Cui Erjia
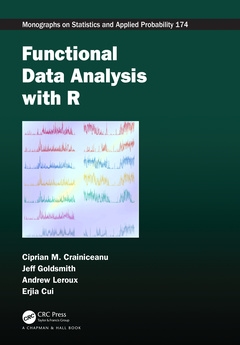
Emerging technologies generate data sets of increased size and complexity that require new or updated statistical inferential methods and scalable, reproducible software. These data sets often involve measurements of a continuous underlying process, and benefit from a functional data perspective. Functional Data Analysis with R presents many ideas for handling functional data including dimension reduction techniques, smoothing, functional regression, structured decompositions of curves, and clustering. The idea is for the reader to be able to immediately reproduce the results in the book, implement these methods, and potentially design new methods and software that may be inspired by these approaches.
Features:
- Functional regression models receive a modern treatment that allows extensions to many practical scenarios and development of state-of-the-art software.
- The connection between functional regression, penalized smoothing, and mixed effects models is used as the cornerstone for inference.
- Multilevel, longitudinal, and structured functional data are discussed with emphasis on emerging functional data structures.
- Methods for clustering functional data before and after smoothing are discussed.
- Multiple new functional data sets with dense and sparse sampling designs from various application areas are presented, including the NHANES linked accelerometry and mortality data, COVID-19 mortality data, CD4 counts data, and the CONTENT child growth study.
- Step-by-step software implementations are included, along with a supplementary website (www.FunctionalDataAnalysis.com) featuring software, data, and tutorials.
- More than 100 plots for visualization of functional data are presented.
Functional Data Analysis with R is primarily aimed at undergraduate, master's, and PhD students, as well as data scientists and researchers working on functional data analysis. The book can be read at different levels and combines state-of-the-art software, methods, and inference. It can be used for self-learning, teaching, and research, and will particularly appeal to anyone who is interested in practical methods for hands-on, problem-forward functional data analysis. The reader should have some basic coding experience, but expertise in R is not required.
1. Basic Concepts. 2. Key Methodological Concepts. 3. Functional Principal Components Analysis. 4. Scalar-on-Function Regression. 5. Function-on-Scalar Regression. 6. Function-on-Function Regression. 7. Survival Analysis with Functional Predictors. 8. Multilevel Functional Data Analysis. 9. Clustering of Functional Data.
Ciprian M. Crainiceanu is Professor of Biostatistics at Johns Hopkins University working on wearable and implantable technology (WIT), signal processing, and clinical neuroimaging. He has extensive experience in mixed effects modeling, semiparametric regression, and functional data analysis with application to data generated by emerging technologies.
Jeff Goldsmith is Associate Dean for Data Science and Associate Professor of Biostatistics at the Columbia University Mailman School of Public Health. His work in functional data analysis includes methodological and computational advances with applications in reaching kinematics, wearable devices, and neuroimaging.
Andrew Leroux is an Assistant Professor of Biostatistics and Informatics at the University of Colorado. His interests include the development of methodology in functional data analysis, particularly related to wearable technologies and intensive longitudinal data.
Erjia Cui is an Assistant Professor of Biostatistics at the University of Minnesota. His research interests include developing functional data analysis methods and semiparametric regression models with reproducible software, with applications in wearable devices, mobile health, and imaging.
Date de parution : 03-2024
17.8x25.4 cm