Regression Modeling Methods, Theory, and Computation with SAS
Auteur : Panik Michael
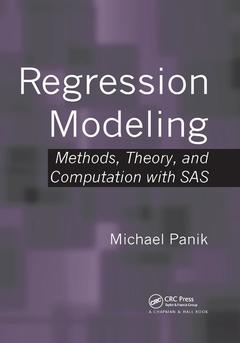
Regression Modeling: Methods, Theory, and Computation with SAS provides an introduction to a diverse assortment of regression techniques using SAS to solve a wide variety of regression problems. The author fully documents the SAS programs and thoroughly explains the output produced by the programs.
The text presents the popular ordinary least squares (OLS) approach before introducing many alternative regression methods. It covers nonparametric regression, logistic regression (including Poisson regression), Bayesian regression, robust regression, fuzzy regression, random coefficients regression, L1 and q-quantile regression, regression in a spatial domain, ridge regression, semiparametric regression, nonlinear least squares, and time-series regression issues. For most of the regression methods, the author includes SAS procedure code, enabling readers to promptly perform their own regression runs.
A Comprehensive, Accessible Source on Regression Methodology and ModelingRequiring only basic knowledge of statistics and calculus, this book discusses how to use regression analysis for decision making and problem solving. It shows readers the power and diversity of regression techniques without overwhelming them with calculations.
Preface. Review of Fundamentals of Statistics. Bivariate Linear Regression and Correlation. Misspecified Disturbance Terms. Nonparametric Regression. Logistic Regression. Bayesian Regression. Robust Regression. Fuzzy Regression. Random Coefficients Regression. L1 and q-Quantile Regression. Regression in a Spatial Domain. Multiple Regression. Normal Correlation Models. Ridge Regression. Indicator Variables. Polynomial Model Estimation. Semiparametric Regression. Nonlinear Regression. Issues in Time Series Modeling and Estimation. Appendix. References. Index.
Date de parution : 10-2019
17.8x25.4 cm
Date de parution : 05-2009
17.8x25.4 cm
Thèmes de Regression Modeling :
Mots-clés :
SAS System; Root MSE; regression modeling; SAS Code; SAS; Source DF Sum; ordinary least squares; DF Parameter Estimate Standard Error; maximum likelihood; OLS Estimator; time series modeling; Variable DF Parameter Estimate Standard; robust regression; Coeff Var; homoscedasticity; Variable DF Parameter Estimate; Proc Reg Data; Regression Model; OLS Estimate; Reg Procedure Model; OLS Regression; Model Statement; Test H0; Proc Print Data; Regressors X1; WLS Estimator; SAS Dataset; Reject H0; Fuzzy Linear Regression Model; ARIMA Procedure; Smoothing Parameter; OLS Residual