Data Mining and Knowledge Discovery for Geoscientists
Auteur : Shi Guangren
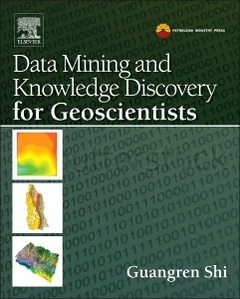
Currently there are major challenges in data mining applications in the geosciences. This is due primarily to the fact that there is a wealth of available mining data amid an absence of the knowledge and expertise necessary to analyze and accurately interpret the same data. Most geoscientists have no practical knowledge or experience using data mining techniques. For the few that do, they typically lack expertise in using data mining software and in selecting the most appropriate algorithms for a given application. This leads to a paradoxical scenario of "rich data but poor knowledge".
The true solution is to apply data mining techniques in geosciences databases and to modify these techniques for practical applications. Authored by a global thought leader in data mining, Data Mining and Knowledge Discovery for Geoscientists addresses these challenges by summarizing the latest developments in geosciences data mining and arming scientists with the ability to apply key concepts to effectively analyze and interpret vast amounts of critical information.
The primary audience includes researchers in data mining, and scientists and engineers in the geosciences. A secondary audience includes scientists and engineers in computer science and information technology, and graduate students taking related coursework.
- Focuses on 22 of data mining’s most practical algorithms and popular application samples
- Features 36 case studies and end-of-chapter exercises unique to the geosciences to underscore key data mining applications
- Presents a practical and integrated system of data mining and knowledge discovery for geoscientists
- Rigorous yet broadly accessible to geoscientists, engineers, researchers and programmers in data mining
- Introduces widely used algorithms, their basic principles and conditions of applications, diverse case studies, and suggests algorithms that may be suitable for specific applications
Date de parution : 12-2013
Ouvrage de 376 p.
19x23.3 cm
Thèmes de Data Mining and Knowledge Discovery for Geoscientists :
Mots-clés :
Bayesian discrimination; Bayesian successive discrimination; Bayesian theorem; C4.5; ID3; Kriging; Monte Carlo method; Q-mode; R-mode; XOR problem; algorithm selection; algorithms running; applications of Kriging; back-propagation neural network (BPNN); classification; classification algorithms; classification rules; cluster analysis; coal gas outburst; computer buy; cross validation; data induction learning; data input; data mining; data mining system; data preprocessing; decision trees; defaulted borrower; dimension reduction; distance weighted; error analysis; experimental variogram; exploration drilling object; faults; fractal geometry; fracture-acidizing prediction; fractures prediction; fuzzy integrated decision; gas layer classification; geosciences particularities; gray integrated decision; gray prediction; least-squares method; linear algorithms; linear programming; multiple regression analysis; naïve Bayesian; nearest point; non-distance weighted; nonlinear algorithms; nonlinearity; nonlinearity ranking; oil layer classification; oil production prediction; optimal fitting; optimal learning time count; optimal profit; pore volume; porosity; preprocessing; probability density function; proved reserve; regression; regression algorithms; reserves in place; reservoir classification; reservoir prediction; results output; sample reduction; software system; solution accuracy ranking; spherical model; support vector machine; system components; top coal caving; trap quality; undiscovered resources; well-logging data; well-logging interpretation; well-logging interpretationm