Granular Computing Based Machine Learning, Softcover reprint of the original 1st ed. 2018 A Big Data Processing Approach Studies in Big Data Series, Vol. 35
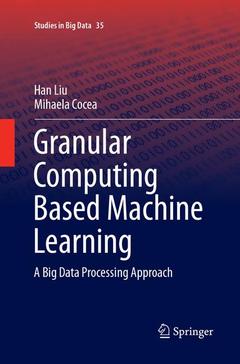
Han Liu is currently a Research Associate in Data Science in the School of Computer Science and Informatics at the Cardiff University. He has previously been a Research Associate in Computational Intelligence in the School of Computing at the University of Portsmouth. He received a BSc in Computing from University of Portsmouth in 2011, an MSc in Software Engineering from University of Southampton in 2012, and a PhD in Machine Learning from University of Portsmouth in 2015.
His research interests include data mining, machine learning, rule based systems, granular computing, intelligent systems, fuzzy systems, big data, computational intelligence and applications in cyber security, cyber crime, cyber bullying, cyber hate and pattern recognition.
He published a research monograph with Springer in the third year of his PhD. He also published over 25 papers in the areas such as data mining, machine learning and granular computing. One of his papers wasidentified as a key scientific article contributing to scientific and engineering research excellence by the selection team at Advances in Engineering and the selection rate is less than 0.1% as indicated. He also has a paper selected as a finalist of Lotfi Zadeh Best Paper Award in the 16th International Conference on Machine Learning and Cybernetics (ICMLC 2017) and has another paper nominated for Lotfi Zadeh Best Paper Award in the 15th International Conference on Machine Learning and Cybernetics (ICMLC 2016).
He has been registered as a reviewer for several established journals, such as IEEE Transactions on Fuzzy Systems, and Information Sciences (Elsevier). He has also recently been a member of the programme committee for the 17th UK Workshop on Computational Intelligence (UKCI 2017), the 16th International Conference on Machine Learning and Cybernetics (ICMLC 2017) and the 2nd IET International Conference on Biomedical Image and Signal Processing (ICBISP 2017). He is
Date de parution : 09-2018
Ouvrage de 113 p.
15.5x23.5 cm
Date de parution : 11-2017
Ouvrage de 113 p.
15.5x23.5 cm