Identification of Dynamical Systems with Small Noise, 1994 Mathematics and Its Applications Series, Vol. 300
Langue : Anglais
Auteur : Kutoyants Yury A.
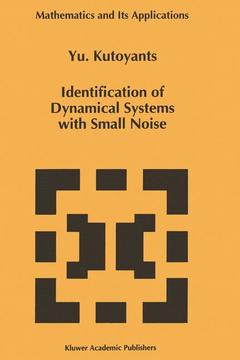
Small noise is a good noise. In this work, we are interested in the problems of estimation theory concerned with observations of the diffusion-type process Xo = Xo, 0 ~ t ~ T, (0. 1) where W is a standard Wiener process and St(') is some nonanticipative smooth t function. By the observations X = {X , 0 ~ t ~ T} of this process, we will solve some t of the problems of identification, both parametric and nonparametric. If the trend S(-) is known up to the value of some finite-dimensional parameter St(X) = St((}, X), where (} E e c Rd , then we have a parametric case. The nonparametric problems arise if we know only the degree of smoothness of the function St(X), 0 ~ t ~ T with respect to time t. It is supposed that the diffusion coefficient c is always known. In the parametric case, we describe the asymptotical properties of maximum likelihood (MLE), Bayes (BE) and minimum distance (MDE) estimators as c --+ 0 and in the nonparametric situation, we investigate some kernel-type estimators of unknown functions (say, StO,O ~ t ~ T). The asymptotic in such problems of estimation for this scheme of observations was usually considered as T --+ 00 , because this limit is a direct analog to the traditional limit (n --+ 00) in the classical mathematical statistics of i. i. d. observations. The limit c --+ 0 in (0. 1) is interesting for the following reasons.
1 Auxiliary Results.- 1.1 Some notions of probability theory.- 1.2 Stochastic integral.- 1.3 On asymptotic estimation theory.- 2 Asymptotic Properties of Estimators in Standard and Nonstandard Situations.- 2.1 LAM bound on the risks of estimators.- 2.2 Asymptotic behavior of estimators in the regular case.- 2.3 Parameter estimation for linear systems.- 2.4 Nondifferentiable and “too differentiable” trends.- 2.5 Random initial value.- 2.6 Misspecified models.- 2.7 Nonconsistent estimation.- 2.8 Boundary of the parametric set.- 3 Expansions.- 3.1 Expansion of the MLE.- 3.2 Possible generalizations.- 3.3 Expansion of the distribution function.- 4 Nonparametric Estimation.- 4.1 Trend estimation.- 4.2 Linear multiplier estimation.- 4.3 State estimation.- 5 The Disorder Problem.- 5.1 Simultaneous estimation of the smooth parameter and the moment of switching.- 5.2 Multidimensional disorder.- 5.3 Misspecified disorder.- 6 Partially Observed Systems.- 6.1 Kalman filter identification.- 6.2 Nonlinear systems.- 6.3 Disorder problem for Kalman filter.- 7 Minimum Distance Estimation.- 7.1 Definitions and examples of the MDE.- 7.2 Consistence and limit distributions.- 7.3 Linear systems.- 7.4 Nonstandard situations and other problems.- 7.5 Asymptotic efficiency of the MDE.- Remarks.- References.
This volume studies parametric and nonparametric estimation through the observation of diffusion-type processes. The properties of maximum likelihood, Bayes, and minimum distance estimators are considered in the context of the asymptotics of low noise. It is shown that, under certain conditions relating to regularity, these estimators are consistent and asymptotically normal. Their properties in nonregular cases are also discussed. Here, nonregularity means the absence of derivatives with respect to parameters, random initial value, incorrectly specified observations, nonidentifiable models, etc.
Date de parution : 10-2012
Ouvrage de 301 p.
16x24 cm
Disponible chez l'éditeur (délai d'approvisionnement : 15 jours).
Prix indicatif 105,49 €
Ajouter au panierThèmes d’Identification of Dynamical Systems with Small Noise :
© 2024 LAVOISIER S.A.S.