Modeling and Optimization in Manufacturing Toward Greener Production by Integrating Computer Simulation
Coordonnateurs : Pruncu Catalin I., Jiang Jun
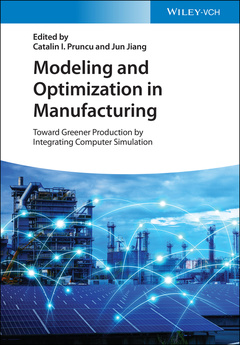
Discover the state-of-the-art in multiscale modeling and optimization in manufacturing from two leading voices in the field
Modeling and Optimization in Manufacturing delivers a comprehensive approach to various manufacturing processes and shows readers how multiscale modeling and optimization processes help improve upon them. The book elaborates on the foundations and applications of computational modeling and optimization processes, as well as recent developments in the field. It offers discussions of manufacturing processes, including forming, machining, casting, joining, coating, and additive manufacturing, and how computer simulations have influenced their development.
Examples for each category of manufacturing are provided in the text, and industrial applications are described for the reader. The distinguished authors also provide an insightful perspective on likely future trends and developments in manufacturing modeling and optimization, including the use of large materials databases and machine learning. Readers will also benefit from the inclusion of:
- A thorough introduction to the origins of manufacturing, the history of traditional and advanced manufacturing, and recent progress in manufacturing
- An exploration of advanced manufacturing and the environmental impact and significance of manufacturing
- Practical discussions of the economic importance of advanced manufacturing
- An examination of the sustainability of advanced manufacturing, and developing and future trends in manufacturing
Perfect for materials scientists, mechanical engineers, and process engineers, Modeling and Optimization in Manufacturing will also earn a place in the libraries of engineering scientists in industries seeking a one-stop reference on multiscale modeling and optimization in manufacturing.
Preface xiii
History of Traditional/Advanced Manufacturing 1
Esther T. Akinlabi, Michael C. Agarana, and Stephen A. Akinlabi
1 Introduction 1
2 Progress in Manufacturing 3
3 Overview of Advanced Manufacturing 5
4 Environmental Impact and Significance 6
5 Economic Importance of Advanced Manufacturing 7
6 Sustainability of Advanced Manufacturing 9
7 Trend of Advanced Manufacturing (AM) 10
8 Summary 11
References 12
1 Modeling and Optimization in Manufacturing by Hydroforming and Stamping 13
Hakim Naceur and Waseem Arif
1.1 Introduction 13
1.2 Recent Advances in Stamping and Hydroforming Simulation 14
1.2.1 Fast Nonlinear Procedures in Stamping and Hydroforming 16
1.2.1.1 Geometrical Mapping Algorithm 17
1.2.1.2 Radial Length Development Algorithm 17
1.2.1.3 Orthogonal Length Unfolding Algorithm 17
1.2.2 Multistage Inverse Method for Stamping and Hydroforming 20
1.2.2.1 Generation of Intermediate Configurations 21
1.2.2.2 Integration of Stress States 22
1.2.2.3 Procedure for Mapping Fields between Two Configurations 22
1.2.2.4 Application to the Demeri Cylindrical Cup 24
1.2.3 Improved Inverse Method for Stamping and Hydroforming 27
1.2.3.1 Basic Idea 27
1.2.3.2 Deformation Path Prediction 27
1.2.3.3 Consideration of Bending Moments 27
1.2.3.4 Bending and Unbending Problem 29
1.2.3.5 Application to the Square Box of Numisheet93 30
1.3 Optimization of Stamping and Hydroforming Parameters 31
1.3.1 Mathematical Optimization Problem 33
1.3.2 Shape Optimization of the Initial Blank 33
1.3.3 Optimization of Addendum Surfaces of Stamped Parts 34
1.3.4 Optimization of Drawbead Restraining Forces 36
1.3.5 Optimization of Tool Geometry 38
1.3.6 Optimization of Material Parameters 39
1.3.7 Optimization of Hydroforming Process Parameters 41
1.4 Future Outlooks 43
1.5 Conclusions 44
References 45
2 Numerical Simulation Techniques in Casting Process 49
Qingyan Xu and Cong Yang
2.1 Introduction 49
2.2 Numerical Models 50
2.2.1 Heat Transfer Model 50
2.2.1.1 Heat Conduction 50
2.2.1.2 Heat Convection 51
2.2.1.3 Heat Radiation 51
2.2.1.4 Heat Conduction Partial Differential Equation 52
2.2.1.5 Finite Difference Method for Solving Heat Transfer Problem 52
2.2.2 Fluid Flow Model 53
2.2.2.1 Continuity Equation 54
2.2.2.2 Navier–Stokes Equation 54
2.2.2.3 Numerical Algorithms 54
2.2.2.4 Free Surface Track 55
2.2.3 Stress Simulation Model 56
2.2.3.1 Thermal Elastoplastic Model 57
2.2.3.2 Numerical Solution 57
2.2.4 Microstructure Simulation Model 58
2.2.4.1 The Nucleation Model 58
2.2.4.2 The Cellular Automaton (CA) Method 59
2.2.4.3 The Phase-Field Method 59
2.2.5 Initial and Boundary Conditions 61
2.2.5.1 Initial Conditions 61
2.2.5.2 Boundary Conditions 61
2.3 Modeling Casting Process and Optimization 62
2.3.1 Mold Filling Simulation 62
2.3.1.1 Cylinder Head Cover Filling Simulation 62
2.3.1.2 Aircraft Cabin Door Casting Simulation 63
2.3.2 Solidification Simulation 64
2.3.2.1 Comparison Study of DS Solidification Simulation 67
2.3.2.2 Processing Parameter Optimization Using Solidification Simulation 69
2.3.3 Stress Simulation 70
2.3.3.1 Hollow Bar Stress Simulation 71
2.3.3.2 Turbine Blade Stress Simulation 71
2.3.4 Casting Microstructure Simulation 74
2.3.4.1 Casting Grain Structure Simulation 74
2.3.4.2 Dendrite Microstructure Simulation 76
2.4 Conclusion 79
Acknowledgments 79
References 80
3 Modeling and Optimization Process in Milling 83
Bogdan A. Chirita
3.1 Milling 83
3.1.1 Introduction 83
3.1.2 Lightweight Alloys Machining 85
3.2 Modeling and Simulation of Milling 86
3.2.1 Response Surface Methodology 87
3.2.1.1 Application of RSM to Machining 88
3.2.1.2 Response Surface Methodology Applied to Investigate the Cutting Force in Face Milling of AZ61A Magnesium Alloy Parts 89
3.2.2 Fuzzy Logic 98
3.2.2.1 Application of Fuzzy Method in Machining 101
3.2.2.2 Example of Fuzzy Logic Applied to Predict Surface Roughness in Magnesium Milling 102
3.3 Conclusion 106
References 106
4 Modeling and Optimization of the Abrasive Water Jet Cutting Process 113
Popan I. Alexandru
4.1 Introduction 113
4.2 Purpose and Methods 115
4.3 Description of Experimental Setup 118
4.4 Modeling and Optimization Process 120
4.4.1 Mathematical Modeling 120
4.4.2 Effect of Machining Parameters on Quality Characteristics 126
4.5 Process Optimization 127
4.6 Conclusions 129
References 130
5 Modeling and Optimization in Manufacturing by Laser 133
Manuela Pacella
5.1 Introduction 133
5.2 Analytical Modeling of Laser Processing 134
5.2.1 Process Parameters in Laser Micromachining 135
5.2.2 Case Study – Topographical Modeling of Laser Micromachining 137
5.2.3 Case Study – Modeling the Recoil Pressure in the Evaporation Stage of Laser Micromachining 140
5.3 Experimental Optimization of Laser Micromachining of Complex 3D Freeform Surfaces 144
5.3.1 Experimental Optimization of Channels/Grooves-like Structures 144
5.3.2 Experimental Optimization of 3D Complex Freeform Surfaces 147
5.4 Experimental Characterization of the Subsurface Integrity of Complex 3D Freeform Surfaces Post Laser Manufacturing 151
5.5 Concluding Remarks 154
References 155
6 Introduction to Isostatic Pressing and Its Optimization 157
Gautam R. Vadolia, K. Premjit Singh, Manoj K. Gupta, Bharat Doshi, and Vikas Rathore
6.1 Introduction 157
6.2 HIP Technology Positioning 159
6.3 HIP Production Process for Powder Metallurgy 159
6.3.1 Melting and Atomization 160
6.3.2 Canning 161
6.3.3 Hot Isostatic Pressing (HIP) Process 163
6.3.3.1 Pressure Vessel 164
6.3.3.2 Furnace 164
6.3.3.3 Gas Handling 165
6.3.3.4 Controls 165
6.3.3.5 Auxiliary Systems 166
6.3.4 Post Processing 166
6.4 Recent Developments in HIP Equipment and HIP Processes 166
6.4.1 Availability of Higher Size Equipment 167
6.4.2 Equipment with Uniform Rapid Cooling (URC) 167
6.4.3 Equipment with Uniform Rapid Quenching (URQ) 168
6.4.4 Gas Impregnation Equipment, Insulation HIP, High-Pressure HIP [50, 55] 168
6.4.5 Sinter-HIP 168
6.4.6 HIP for Nuclear Waste Treatment 169
6.4.7 Preheating HIP Equipment 170
6.4.8 Atmosphere Control HIP Treatment 170
6.4.9 Densal HIP Process 170
6.4.10 Liquid HIP (LHIP) 171
6.5 HIP Applications 171
6.5.1 Densification of Product 172
6.5.2 Consolidation of Powder for Near Net Shape (NNS) 173
6.5.3 Cladding and Diffusion Bonding 174
6.6 Typical HIP Installations 175
6.7 Optimization in HIP 176
6.7.1 Optimization of Process Parameters 177
6.7.2 Optimization of Container/Canister Size 179
6.7.2.1 Macroscopic Approach 179
6.7.2.2 Microscopic Approach 182
6.7.2.3 Soft Computing 183
6.8 Summary 184
References 186
7 Modeling and Optimization Algorithms in Rapid Prototyping, Submerged Arc Welding, and Turning 193
Munish K. Gupta, Mozammel Mia, Nancy Gupta, Sunpreet Singh, Ankush Choudhary, Muhammad Jamil, Aqib M. Khan, Krzysztof Nadolny, Wojciech Kapłonek, Danil Y. Pimenov, and Catalin I. Pruncu
7.1 Introduction 193
7.2 Evolutionary Algorithms 195
7.2.1 Particle Swarm Optimization 195
7.2.2 Classical Bacteria Foraging Optimization 196
7.2.3 Self-Adaptive Bacteria Foraging Optimization 197
7.2.4 Invasive Weed Optimization 198
7.2.5 Artificial Bee Colony Optimization 198
7.3 Experimental Conditions 199
7.3.1 Fused Deposition Modeling 199
7.3.1.1 Technical Specifications for FDM 200
7.3.1.2 Optimization Model for FDM 200
7.3.2 Submerged Arc Welding 201
7.3.2.1 Technical Specifications for SAW 201
7.3.2.2 Optimization Model for SAW 202
7.3.3 Turning of Inconel-800 Alloy 202
7.3.3.1 Technical Specifications for Turning 203
7.3.3.2 Optimization Model for Turning 203
7.4 Results and Discussion 203
7.4.1 Parameters Initialization for Proposed Algorithms 203
7.4.2 Optimization 206
7.4.2.1 Optimization of FDM Parameters 206
7.4.2.2 Optimization of SAW Parameters 207
7.4.2.3 Optimization of Turning Parameters 209
7.5 Best Optimization Model 210
7.6 Conclusions 210
Compliance with Ethical Standards 211
References 211
8 Optimization of Deposition Parameters in Plasma Spray Coatings 217
Keshavamurthy Ramaiah, Naveena Bettahalli Eswaregowda, Vijay Tambrallimath, and Prabhakar Kuppahalli
8.1 Introduction 217
8.1.1 Effect of Plasma Spray Process Parameters 220
8.1.1.1 Plasma Power 221
8.1.1.2 Plasma Gas 222
8.1.1.3 Carrier Gas 222
8.1.1.4 Mass Flow Rate of Powder 223
8.1.1.5 Stand-Off-Distance 224
8.1.1.6 Spraying Angle 225
8.1.1.7 Angle of Powder Injection 225
8.1.2 Powder-Related Variables 225
8.1.2.1 Substrate-Related Variables 227
8.2 APS Process Optimization and Modeling 228
8.3 Case Study 230
8.4 Conclusions 231
References 232
9 Modeling and Optimization Methods in Forming Processes 237
Catalin I. Pruncu, Jun Jiang, and Jianguo Lin
9.1 Introduction 237
9.2 Materials Used in Metal Forming Processes 238
9.3 Sheet Manufacturing 239
9.4 Metal Forming Challenges 239
9.4.1 Friction in Metal Forming 243
9.4.2 Tool Wear of Metal Forming 245
9.4.3 Coatings in Metal Forming 246
9.4.4 Micromechanics Modeling of Micro-Forming Processes 247
9.5 Conclusions 247
References 248
10 Advances in Manufacturing, Laser Additive Techniques: Case Study 253
Esther T. Akinlabi, Michael C. Agarana, and Stephen A. Akinlabi
10.1 Introduction 253
10.2 Advanced Manufacturing Technology 262
10.2.1 Additive Manufacturing 262
10.2.1.1 Electron Beam 263
10.2.1.2 Laser-Based AM Process 263
10.2.2 Advances in Additive Manufacturing 264
10.2.3 Future Trends in Additive Manufacturing 265
10.2.3.1 Robotics 265
10.2.3.2 Manufacturing in the 4th Industrial Revolution 266
10.2.3.3 Modeling in Manufacturing 267
10.3 Applications of Laser Additive Techniques 268
10.3.1 Application to Aerospace 268
10.3.2 Application to Medicine 270
10.3.3 Application to Fine Arts 271
10.4 Sustainability of Advanced Manufacturing Techniques 273
10.4.1 Sustainability of Process and Equipment 273
10.4.2 Sustainability of Applications 275
10.4.2.1 Advantages 276
10.4.2.2 Disadvantages 276
10.4.3 Comparative Advantage Sustainability 277
10.5 Mathematical Methods for Advanced Manufacturing 279
10.5.1 Mathematical Techniques 279
10.5.2 Operational Research Techniques 280
10.5.2.1 Applications of Waiting Line Theory 281
10.5.2.2 Application of Game Theory 281
10.5.2.3 Simulation and Monte Carlo Technique 282
10.5.2.4 Dynamic Programming 283
10.5.3 Heuristic Techniques 284
10.5.4 Statistical Techniques 284
10.6 Theoretical Analysis of Laser Additive Manufacturing 284
10.6.1 Modeling and Simulation of Laser Additive Manufacturing 284
10.6.2 Future Research Direction in Theoretical Additive Manufacturing 288
10.7 Technology Challenges 288
10.7.1 Challenges in Advanced Manufacturing 288
10.7.2 Challenges in Laser Additive Manufacturing 290
10.7.3 Suggested Solutions to Technology Challenges 293
10.8 Summary 294
References 295
Index 303
Catalin I. Pruncu, PhD, is Research Associate in the Department of Mechanical Engineering at Imperial College in London, United Kingdom. He received his doctorate in Design Mechanics and Biomechanics from Politecnico di Bari in Italy in 2013.
Jun Jiang, PhD, is a Lecturer of Mechanics of Materials Division in the Department of Mechanical Engineering at Imperial College London, UK. He received his DPhil from Oxford University in 2013 and joined Imperial College as postdoctoral researcher. Dr. Jiang’s research focuses on developing novel manufacturing techniques through the understanding of micro-thermomechanical behaviors for lightweight alloys and solar cells.
Date de parution : 07-2021
Ouvrage de 336 p.
17x24.4 cm