Towards User-Centric Intelligent Network Selection in 5G Heterogeneous Wireless Networks, 1st ed. 2020 A Reinforcement Learning Perspective
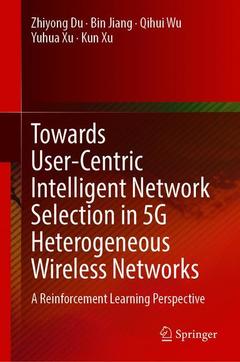
Introduction.- Learning the Optimal Network with Handoff Constraint: MAB RL Based Network Selection.- Learning the Optimal Network with Context Awareness: Transfer RL Based Network Selection.- Meeting Dynamic User Demand with Transmission Cost Awareness: CT-MAB RL Based Network Selection.- Meeting Dynamic User Demand with Handoff Cost Awareness: MDP RL Based Network Handoff.- Matching Heterogeneous User Demands: Localized Cooperation Game and MARL based Network Selection.- Exploiting User Demand Diversity: QoE game and MARL Based Network Selection.- Future Work.
Zhiyong Du received his B.S. degree in Electronic Information Engineering from Wuhan University of Technology, Wuhan, China, in 2009, and his Ph.D. degree in Communications and Information Systems from the College of Communications Engineering, PLA University of Science and Technology, Nanjing, China, in 2015. He is currently a lecturer at the National University of Defense Technology. His research interests include 5G, quality of experience (QoE), learning theory, and game theory.
Bin Jiang received his B.S. degree in Communication Engineering and Ph.D. degree in Information and Communication Engineering both from the National University of Defense Technology, Changsha, China, in 1996 and 2006, respectively. He is currently a Professor at the National University of Defense Technology. His research interests include 5G, artificial intelligence, and wireless signal processing.
Qihui Wu received his B.S., M.S., and Ph.D. degrees in Communications and Information Systems from the PLA University of Science and Technology, Nanjing, China, in 1994, 1997, and 2000, respectively. He is Professor at the College of Electronic and Information Engineering, Nanjing University of Aeronautics and Astronautics. His current research interests include algorithms and optimization for cognitive wireless networks, software-defined radio, and wireless communication systems.
Yuhua Xu received his B.S. degree in Communication Engineering and Ph.D. degree in Communications and Information Systems from the College of Communications Engineering, PLA University of Science and Technology, in 2006 and 2014, respectively. He is currently an Associate Professor at the College of Communications Engineering, Army Engineering University of PLA. He has published several papers in international conferences and respected journals. His research interests include UAV communication networks, opportunistic spectrum access, learning theory, and distributed optimization techniques for wi
Date de parution : 11-2019
Ouvrage de 136 p.
15.5x23.5 cm
Date de parution : 11-2020
Ouvrage de 136 p.
15.5x23.5 cm