Social Big Data Analytics, 1st ed. 2021 Practices, Techniques, and Applications
Auteurs : Abu-Salih Bilal, Wongthongtham Pornpit, Zhu Dengya, Chan Kit Yan, Rudra Amit
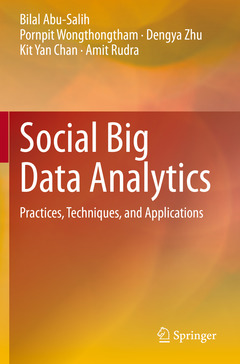
Big data is no more “all just hype” but widely applied in nearly all aspects of our business, governments, and organizations with the technology stack of AI. Its influences are far beyond a simple technique innovation but involves all rears in the world. This chapter will first have historical review of big data; followed by discussion of characteristics of big data, i.e. the 3V’s to up 10V’s of big data. The chapter then introduces technology stacks for an organization to build a big data application, from infrastructure/platform/ecosystem to constructional units/components; following by several successful examples. Finally, we provide some big data online resources for reference.
Chapter 2: Credibility and influence in social big data
Online Social Networks (OSNs) are a fertile medium through which users can express their sentiments and share their opinions, experiences and knowledge of several topics. There is a deficiency of assessment mechanisms that incorporate domain-based trustworthiness. In OSNs, determining users’ influence in a particular domain has been driven by its significance in a broad range of applications such as personalized recommendation systems, opinion analysis, expertise retrieval, to name a few. This chapter presents a comprehensive framework that aims to infer value from BSD by measuring the domain-based trustworthiness of OSN users, addressing the main features of big data, and incorporating semantic analysis and the temporal factor.
Chapter 3: Semantic data discovery from social big data
The challenge of managing and extracting useful knowledge from social media data sources has attracted much attention from academia and industry. Social big data is an important big data island; thus, social data analytics are intended to make sense of data and to obtain value from data. Social big data provides a wealth of information that businesses, political governments, organisations, etc. can mine and analyse to exploit value in a variety of areas. This chapter discusses the development of an approach that aims to semantically analyse social content, thus enriching social data with semantic conceptual representation for domain-based discovery.
Chapter 4: Predictive analytics using social big data and machine learning
Previous works in the area of topic distillation and discovery lack an appropriate and applicable technical solution that can handle the complex task of obtaining an accurate interpretation of the contextual social content. This is evident through the inadequacy of these endeavours in addressing the topics of microblogging short messages like tweets, and their inability to classify and predict the messages’ actual and precise domains of interest at the user level. Hence, this chapter intends to address this problem by presenting solutions to domain-based classification and prediction of social big data at the user and tweet levels incorporating comprehensive knowledge discovery tools and well-known machine learning algorithms.
Chapter 5: Affective design in the era of big social data
In today’s competitive market, product designers not only need to optimize functional qualities when developing a new product, but also they need to optimize the affective qualities of the product. The reason is that products with high affective qualities is more likely to attract more potential consumers to buy. In the past, affective design is generally conducted based on the limited amount of customer survey data which is collected from marketing questionnaires and consumer interviews. Since the data amount is limited, the affective design cannot fully reflect the current or even the recent situation of the marketplaces. Thanks to the advanced computing and web technologies, big data from social media or product reviews in web can be used to conduct affective design. Computational technologies involved with big data analysis are recently more popular to be implemented on affective design. This chapter reviews computational techniques for affective design. The reviews are based on two main streams of data namely small data which is collected from customer survey and big data collected from social media or product reviews in web. The limitations and advantages are discussed when these two main streams of data are implemented. The challenges of implementing big data for affective design are also discussed.
Chapter 6: Social sentimental analysis
Sentiment Analysis (a.k.s Opinion mining) intends to discover public opinions, sentiments towards other entities. This chapter introduces how to apply big data technology to keep the track of sentiments/opinions of public news media on give topics such as real-estate market in Australia. We first describe the big data framework, a Hadoop cluster, employed in our project. Followed by a detailed description of approaches and models utilized in our research; experimental design, and experiment data set statistics. We presented our experimental results with a list of tables and figures to demonstrate how big data techniques can successfully illustrate news media’s sentiments towards the Australia real-estate market from different angles based on our big data set collected from the Web.
Dr Bilal Abu Salih holds a Ph.D. in Information Systems with a focus on Social Big Data Analytics from Curtin University since 2018. He is an Assistant Professor in the Computer Science Department in King Abdullah II School of Information Technology, The University of Jordan, Jordan. Bilal also a University Associate, Curtin University, Australia. He worked with cross-disciplinary funded research projects which are related to data analytics, machine learning, data mining of social media, and big data analysis.
Dr Pornpit Wongthongtham aka Dr Ponnie Clark is the recipient of a PhD in Information Systems, Master of Science in Computer Science, and Bachelor of Science in Mathematics. Her research areas of expertise include Ontology and Linked Data, Text Mining, Social Data Analytics, Trustworthiness, Artificial Intelligence, and the like. She has over two decades of academic experience including in research and development involving multi-disciplinary areas. She is currently a senior research fellow at School of Physics, Maths and Computing, the University of Western Australia.
Dr Dengya (Simon) Zhu is an adjunct research fellow at the School of Management, Curtin Business School. He has a broad range of experience in government, industry, and academic research. Dr Zhu’s research interests include big data, data mining, machine learning, natural language processing, information retrieval, sentiment analysis, open-source software, and software development. His research projects are usually practically oriented to address real-world issues.
Kit Yan Chan received his Ph.D. degree in Computing in 2006 from London South Bank University, United Kingdom, and is currently a Senior Lecturer at Curtin University, Australia. Dr Kit Yan Chan has worked as a Full-Time Researcher at Hong Kong Polytechnic University (2004–2009) and Curtin University (2009–2013). Dr Kit Yan Chan is an Associate Editor for IJFS, and the editorial board of t
Provides in depth explanation and analysis of Big Social Data
Demonstrates core concepts with many visual illustrations such as tables, graphs and charts
Brings together expert evaluations in Big Social Data, Text Mining, Social Data Analytics, Data Mining and Artificial Intelligence
Date de parution : 03-2022
Ouvrage de 218 p.
15.5x23.5 cm
Date de parution : 03-2021
Ouvrage de 218 p.
15.5x23.5 cm