Forecast Error Correction using Dynamic Data Assimilation, Softcover reprint of the original 1st ed. 2017 Springer Atmospheric Sciences Series
Auteurs : Lakshmivarahan Sivaramakrishnan, Lewis John M., Jabrzemski Rafal
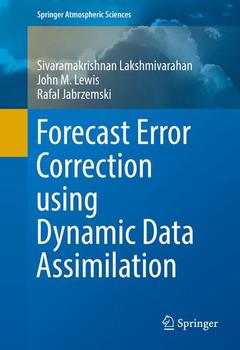
Dr. Sivaramakrishnan Lakshmivarahan (Varahan, for short) joined the University of Oklahoma in the fall of 1978 where he is currently a George Lynn Cross Research Professor at the School of Computer Science. From 1973-1978 he has held postdoctoral and faculty positions at Brown University, Yale University and Indian Institute of Technology, Madras, India. He obtained his PhD in 1973 from the Indian Institute of Science in Bangalore, India. He is the author/coauthor of 5 books and has published extensively in a number of areas including Learning Algorithms, Parallel Architecture and Algorithms, Dynamic Data Assimilation and Computational Finance. He has supervised 30 PhD dissertations and 42 MS Theses. He is Fellow of the IEEE and a Fellow of the ACM and has won numerous awards- Regents award for Superior Teaching and Regents Award for Research and Creative Activity. He has held short-term visiting appointments at leading academic centers in Japan, Taiwan, China, Germany, England, Canada, Mexico and USA. He has been inducted into the Oklahoma Higher Education Hall of Fame in 2014.
John Lewis’ scientific training is in geophysics and meteorology along with an interest and training in applied mathematics, especially mathematical physics with concentration in variational mechanics. Dr. Lewis was fortunate to be trained under George Platzman at University of Chicago and Yoshikazu Sasaki at University of Oklahoma and he has worked for private industry (Shell Oil Co., exploration seismology), operational weather prediction centers (U. S. government and U. S. Navy), academia (University of Illinois), and government science (as a director of research and as a research meteorologist). In the early 1990s, Dr. Lewis came under the influence of historian of science Duane Roller and began contributing to the history of science along with other work in meteorology. He has supervised doctoral dissertations of 10 students, contributed to over 150 resear
Date de parution : 11-2016
Ouvrage de 270 p.
15.5x23.5 cm
Date de parution : 06-2018
Ouvrage de 270 p.
15.5x23.5 cm