Data-Driven Computational Methods Parameter and Operator Estimations
Langue : Anglais
Auteur : Harlim John
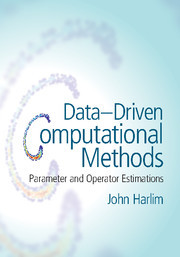
Describes computational methods for parametric and nonparametric modeling of stochastic dynamics. Aimed at graduate students, and suitable for self-study.
Modern scientific computational methods are undergoing a transformative change; big data and statistical learning methods now have the potential to outperform the classical first-principles modeling paradigm. This book bridges this transition, connecting the theory of probability, stochastic processes, functional analysis, numerical analysis, and differential geometry. It describes two classes of computational methods to leverage data for modeling dynamical systems. The first is concerned with data fitting algorithms to estimate parameters in parametric models that are postulated on the basis of physical or dynamical laws. The second is on operator estimation, which uses the data to nonparametrically approximate the operator generated by the transition function of the underlying dynamical systems. This self-contained book is suitable for graduate studies in applied mathematics, statistics, and engineering. Carefully chosen elementary examples with supplementary MATLAB® codes and appendices covering the relevant prerequisite materials are provided, making it suitable for self-study.
1. Introduction; 2. Markov chain Monte Carlo; 3. Ensemble Kalman filters; 4. Stochastic spectral methods; 5. Karhunen–Loève expansion; 6. Diffusion forecast; Appendix A. Elementary probability theory; Appendix B. Stochastic processes; Appendix C. Elementary differential geometry; References; Index.
John Harlim is a Professor of Mathematics and Meteorology at the Pennsylvania State University. His research interests include data assimilation and stochastic computational methods. In 2012, he received the Frontiers in Computational Physics award from the Journal of Computational Physics for his research contributions on computational methods for modeling Earth systems. He has previously co-authored another book, Filtering Complex Turbulent Systems (Cambridge, 2012).
Date de parution : 07-2018
Ouvrage de 168 p.
17.8x25.3 cm
Thème de Data-Driven Computational Methods :
© 2024 LAVOISIER S.A.S.