Advances in Bias and Fairness in Information Retrieval, 1st ed. 2021 Second International Workshop on Algorithmic Bias in Search and Recommendation, BIAS 2021, Lucca, Italy, April 1, 2021, Proceedings Communications in Computer and Information Science Series, Vol. 1418
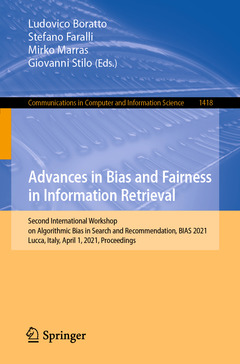
Towards Fairness-Aware Ranking by Defining Latent Groups Using Inferred Features.- Media Bias Everywhere? A Vision for Dealing with the Manipulation of Public Opinion.- Users' Perception of Search-Engine Biases and Satisfaction.- Preliminary Experiments to Examine the Stability of Bias-Aware Techniques.- Detecting Race and Gender Bias in Visual Representation of AI on Web Search Engines.- Equality of Opportunity in Ranking: A Fair-Distributive Model.- Incentives for Item Duplication under Fair Ranking Policies.- Quantification of the Impact of Popularity Bias in Multi-Stakeholder and Time-Aware Environment.- When is a Recommendation Model Wrong? A Model-Agnostic Tree-Based Approach to Detecting Biases in Recommendations.- Evaluating Video Recommendation Bias on YouTube.- An Information-Theoretic Measure for Enabling Category Exemptions with an Application to Filter Bubbles.- Perception-Aware Bias Detection for Query Suggestions.- Crucial Challenges in Large-Scale Black Box Analyses.- New Performance Metrics for Offline Content-based TV Recommender Systems.
Date de parution : 06-2021
Ouvrage de 171 p.
15.5x23.5 cm
Thème d’Advances in Bias and Fairness in Information Retrieval :
Mots-clés :
Computer Science; Informatics; Conference Proceedings; Research; Applications