Uncertainty Modeling for Data Mining, 2014 A Label Semantics Approach Advanced Topics in Science and Technology in China Series
Auteurs : Qin Zengchang, Tang Yongchuan
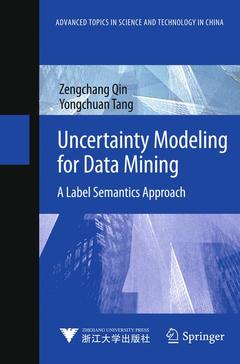
Machine learning and data mining are inseparably connected with uncertainty. The observable data for learning is usually imprecise, incomplete or noisy. Uncertainty Modeling for Data Mining: A Label Semantics Approach introduces 'label semantics', a fuzzy-logic-based theory for modeling uncertainty. Several new data mining algorithms based on label semantics are proposed and tested on real-world datasets. A prototype interpretation of label semantics and new prototype-based data mining algorithms are also discussed. This book offers a valuable resource for postgraduates, researchers and other professionals in the fields of data mining, fuzzy computing and uncertainty reasoning.
Zengchang Qin is an associate professor at the School of Automation Science and Electrical Engineering, Beihang University, China; Yongchuan Tang is an associate professor at the College of Computer Science, Zhejiang University, China.
A new research direction of fuzzy set theory in data mining
One of the first monographs of studying the transparency of data mining models
Contains more than 60 figures and illustrations in order to explain complicated concepts
Date de parution : 03-2014
Ouvrage de 291 p.
15.5x23.5 cm
Disponible chez l'éditeur (délai d'approvisionnement : 15 jours).
Prix indicatif 105,49 €
Ajouter au panier