Trust-based Collective View Prediction, 2013
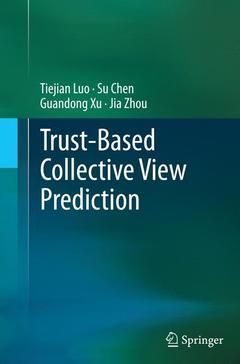
Collective view prediction is to judge the opinions of an active web user based on unknown elements by referring to the collective mind of the whole community. Content-based recommendation and collaborative filtering are two mainstream collective view prediction techniques. They generate predictions by analyzing the text features of the target object or the similarity of users? past behaviors. Still, these techniques are vulnerable to the artificially-injected noise data, because they are not able to judge the reliability and credibility of the information sources. Trust-based Collective View Prediction describes new approaches for tackling this problem by utilizing users? trust relationships from the perspectives of fundamental theory, trust-based collective view prediction algorithms and real case studies.
The book consists of two main parts ? a theoretical foundation and an algorithmic study. The first part will review several basic concepts and methods related to collective view prediction, such as state-of-the-art recommender systems, sentimental analysis, collective view, trust management, the Relationship of Collective View and Trustworthy, and trust in collective view prediction. In the second part, the authors present their models and algorithms based on a quantitative analysis of more than 300 thousand users? data from popular product-reviewing websites. They also introduce two new trust-based prediction algorithms, one collaborative algorithm based on the second-order Markov random walk model, and one Bayesian fitting model for combining multiple predictors.
The discussed concepts, developed algorithms, empirical results, evaluation methodologies and the robust analysis framework described in Trust-based Collective View Prediction will not only provide valuable insights and findings to related research communities and peers, but also showcase the great potential to encourage industries and business partners tointegrate these techniques into new applications.
Preface.- Introduction.- Related Work.- Collaborative Filtering.- Sentiment Analysis.- Theory Foundations.- Models, Methods and Algorithms.- Framework for Robustness Analysis.- Conclusions.- Appendix.
Date de parution : 08-2015
Ouvrage de 146 p.
15.5x23.5 cm
Disponible chez l'éditeur (délai d'approvisionnement : 15 jours).
Prix indicatif 105,49 €
Ajouter au panierDate de parution : 06-2013
Ouvrage de 146 p.
15.5x23.5 cm
Mots-clés :
collective view; data mining; recommender systems; trust management; trust network