Sampling and Estimation from Finite Populations Wiley Series in Survey Methodology Series
Auteur : Tille Yves
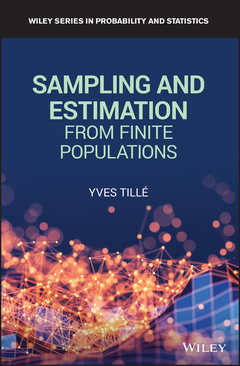
A much-needed reference on survey sampling and its applications that presents the latest advances in the field
Seeking to show that sampling theory is a living discipline with a very broad scope, this book examines the modern development of the theory of survey sampling and the foundations of survey sampling. It offers readers a critical approach to the subject and discusses putting theory into practice. It also explores the treatment of non-sampling errors featuring a range of topics from the problems of coverage to the treatment of non-response. In addition, the book includes real examples, applications, and a large set of exercises with solutions.
Sampling and Estimation from Finite Populations begins with a look at the history of survey sampling. It then offers chapters on: population, sample, and estimation; simple and systematic designs; stratification; sampling with unequal probabilities; balanced sampling; cluster and two-stage sampling; and other topics on sampling, such as spatial sampling, coordination in repeated surveys, and multiple survey frames. The book also includes sections on: post-stratification and calibration on marginal totals; calibration estimation; estimation of complex parameters; variance estimation by linearization; and much more.
- Provides an up-to-date review of the theory of sampling
- Discusses the foundation of inference in survey sampling, in particular, the model-based and design-based frameworks
- Reviews the problems of application of the theory into practice
- Also deals with the treatment of non sampling errors
Sampling and Estimation from Finite Populations is an excellent book for methodologists and researchers in survey agencies and advanced undergraduate and graduate students in social science, statistics, and survey courses.
List of Figures xiii
List of Tables xvii
List of Algorithms xix
Preface xxi
Preface to the First French Edition xxiii
Table of Notations xxv
1 A History of Ideas in Survey Sampling Theory 1
1.1 Introduction 1
1.2 Enumerative Statistics During the 19th Century 2
1.3 Controversy on the use of Partial Data 4
1.4 Development of a Survey Sampling Theory 5
1.5 The US Elections of 1936 6
1.6 The Statistical Theory of Survey Sampling 6
1.7 Modeling the Population 8
1.8 Attempt to a Synthesis 9
1.9 Auxiliary Information 9
1.10 Recent References and Development 10
2 Population, Sample, and Estimation 13
2.1 Population 13
2.2 Sample 14
2.3 Inclusion Probabilities 15
2.4 Parameter Estimation 17
2.5 Estimation of a Total 18
2.6 Estimation of a Mean 19
2.7 Variance of the Total Estimator 20
2.8 Sampling with Replacement 22
Exercises 24
3 Simple and Systematic Designs 27
3.1 Simple Random Sampling without Replacement with Fixed Sample Size 27
3.1.1 Sampling Design and Inclusion Probabilities 27
3.1.2 The Expansion Estimator and its Variance 28
3.1.3 Comment on the Variance–Covariance Matrix 31
3.2 Bernoulli Sampling 32
3.2.1 Sampling Design and Inclusion Probabilities 32
3.2.2 Estimation 34
3.3 Simple Random Sampling with Replacement 36
3.4 Comparison of the Designs with and Without Replacement 38
3.5 Sampling with Replacement and Retaining Distinct Units 38
3.5.1 Sample Size and Sampling Design 38
3.5.2 Inclusion Probabilities and Estimation 41
3.5.3 Comparison of the Estimators 44
3.6 Inverse Sampling with Replacement 45
3.7 Estimation of Other Functions of Interest 47
3.7.1 Estimation of a Count or a Proportion 47
3.7.2 Estimation of a Ratio 48
3.8 Determination of the Sample Size 50
3.9 Implementation of Simple Random Sampling Designs 51
3.9.1 Objectives and Principles 51
3.9.2 Bernoulli Sampling 51
3.9.3 Successive Drawing of the Units 52
3.9.4 Random Sorting Method 52
3.9.5 Selection–Rejection Method 53
3.9.6 The Reservoir Method 54
3.9.7 Implementation of Simple Random Sampling with Replacement 56
3.10 Systematic Sampling with Equal Probabilities 57
3.11 Entropy for Simple and Systematic Designs 58
3.11.1 Bernoulli Designs and Entropy 58
3.11.2 Entropy and Simple Random Sampling 60
3.11.3 General Remarks 61
Exercises 61
4 Stratification 65
4.1 Population and Strata 65
4.2 Sample, Inclusion Probabilities, and Estimation 66
4.3 Simple Stratified Designs 68
4.4 Stratified Design with Proportional Allocation 70
4.5 Optimal Stratified Design for the Total 71
4.6 Notes About Optimality in Stratification 74
4.7 Power Allocation 75
4.8 Optimality and Cost 76
4.9 Smallest Sample Size 76
4.10 Construction of the Strata 77
4.10.1 General Comments 77
4.10.2 Dividing a Quantitative Variable in Strata 77
4.11 Stratification Under Many Objectives 79
Exercises 80
5 Sampling with Unequal Probabilities 83
5.1 Auxiliary Variables and Inclusion Probabilities 83
5.2 Calculation of the Inclusion Probabilities 84
5.3 General Remarks 85
5.4 Sampling with Replacement with Unequal Inclusion Probabilities 86
5.5 Nonvalidity of the Generalization of the Successive Drawing without Replacement 88
5.6 Systematic Sampling with Unequal Probabilities 89
5.7 Deville’s Systematic Sampling 91
5.8 Poisson Sampling 92
5.9 Maximum Entropy Design 95
5.10 Rao–Sampford Rejective Procedure 98
5.11 Order Sampling 100
5.12 Splitting Method 101
5.12.1 General Principles 101
5.12.2 Minimum Support Design 103
5.12.3 Decomposition into Simple Random Sampling Designs 104
5.12.4 Pivotal Method 107
5.12.5 Brewer Method 109
5.13 Choice of Method 110
5.14 Variance Approximation 111
5.15 Variance Estimation 114
Exercises 115
6 Balanced Sampling 119
6.1 Introduction 119
6.2 Balanced Sampling: Definition 120
6.3 Balanced Sampling and Linear Programming 122
6.4 Balanced Sampling by Systematic Sampling 123
6.5 Methode of Deville, Grosbras, and Roth 124
6.6 Cube Method 125
6.6.1 Representation of a Sampling Design in the form of a Cube 125
6.6.2 Constraint Subspace 126
6.6.3 Representation of the Rounding Problem 127
6.6.4 Principle of the Cube Method 130
6.6.5 The Flight Phase 130
6.6.6 Landing Phase by Linear Programming 133
6.6.7 Choice of the Cost Function 134
6.6.8 Landing Phase by Relaxing Variables 135
6.6.9 Quality of Balancing 135
6.6.10 An Example 136
6.7 Variance Approximation 137
6.8 Variance Estimation 140
6.9 Special Cases of Balanced Sampling 141
6.10 Practical Aspects of Balanced Sampling 141
Exercise 142
7 Cluster and Two-stage Sampling 143
7.1 Cluster Sampling 143
7.1.1 Notation and Definitions 143
7.1.2 Cluster Sampling with Equal Probabilities 146
7.1.3 Sampling Proportional to Size 147
7.2 Two-stage Sampling 148
7.2.1 Population, Primary, and Secondary Units 149
7.2.2 The Expansion Estimator and its Variance 151
7.2.3 Sampling with Equal Probability 155
7.2.4 Self-weighting Two-stage Design 156
7.3 Multi-stage Designs 157
7.4 Selecting Primary Units with Replacement 158
7.5 Two-phase Designs 161
7.5.1 Design and Estimation 161
7.5.2 Variance and Variance Estimation 162
7.6 Intersection of Two Independent Samples 163
Exercises 165
8 Other Topics on Sampling 167
8.1 Spatial Sampling 167
8.1.1 The Problem 167
8.1.2 Generalized Random Tessellation Stratified Sampling 167
8.1.3 Using the Traveling Salesman Method 169
8.1.4 The Local Pivotal Method 169
8.1.5 The Local Cube Method 169
8.1.6 Measures of Spread 170
8.2 Coordination in Repeated Surveys 172
8.2.1 The Problem 172
8.2.2 Population, Sample, and Sample Design 173
8.2.3 Sample Coordination and Response Burden 174
8.2.4 Poisson Method with Permanent Random Numbers 175
8.2.5 Kish and Scott Method for Stratified Samples 176
8.2.6 The Cotton and Hesse Method 176
8.2.7 The Rivière Method 177
8.2.8 The Netherlands Method 178
8.2.9 The Swiss Method 178
8.2.10 Coordinating Unequal Probability Designs with Fixed Size 181
8.2.11 Remarks 181
8.3 Multiple Survey Frames 182
8.3.1 Introduction 182
8.3.2 Calculating Inclusion Probabilities 183
8.3.3 Using Inclusion Probability Sums 184
8.3.4 Using a Multiplicity Variable 185
8.3.5 Using a Weighted Multiplicity Variable 186
8.3.6 Remarks 187
8.4 Indirect Sampling 187
8.4.1 Introduction 187
8.4.2 Adaptive Sampling 188
8.4.3 Snowball Sampling 188
8.4.4 Indirect Sampling 189
8.4.5 The Generalized Weight Sharing Method 190
8.5 Capture–Recapture 191
9 Estimation with a Quantitative Auxiliary Variable 195
9.1 The Problem 195
9.2 Ratio Estimator 196
9.2.1 Motivation and Definition 196
9.2.2 Approximate Bias of the Ratio Estimator 197
9.2.3 Approximate Variance of the Ratio Estimator 198
9.2.4 Bias Ratio 199
9.2.5 Ratio and Stratified Designs 199
9.3 The Difference Estimator 201
9.4 Estimation by Regression 202
9.5 The Optimal Regression Estimator 204
9.6 Discussion of the Three Estimation Methods 205
Exercises 208
10 Post-Stratification and Calibration on Marginal Totals 209
10.1 Introduction 209
10.2 Post-Stratification 209
10.2.1 Notation and Definitions 209
10.2.2 Post-Stratified Estimator 211
10.3 The Post-Stratified Estimator in Simple Designs 212
10.3.1 Estimator 212
10.3.2 Conditioning in a Simple Design 213
10.3.3 Properties of the Estimator in a Simple Design 214
10.4 Estimation by Calibration on Marginal Totals 217
10.4.1 The Problem 217
10.4.2 Calibration on Marginal Totals 218
10.4.3 Calibration and Kullback–Leibler Divergence 220
10.4.4 Raking Ratio Estimation 221
10.5 Example 221
Exercises 224
11 Multiple Regression Estimation 225
11.1 Introduction 225
11.2 Multiple Regression Estimator 226
11.3 Alternative Forms of the Estimator 227
11.3.1 Homogeneous Linear Estimator 227
11.3.2 Projective Form 228
11.3.3 Cosmetic Form 228
11.4 Calibration of the Multiple Regression Estimator 229
11.5 Variance of the Multiple Regression Estimator 230
11.6 Choice of Weights 231
11.7 Special Cases 231
11.7.1 Ratio Estimator 231
11.7.2 Post-stratified Estimator 231
11.7.3 Regression Estimation with a Single Explanatory Variable 233
11.7.4 Optimal Regression Estimator 233
11.7.5 Conditional Estimation 235
11.8 Extension to Regression Estimation 236
Exercise 236
12 Calibration Estimation 237
12.1 Calibrated Methods 237
12.2 Distances and Calibration Functions 239
12.2.1 The Linear Method 239
12.2.2 The Raking Ratio Method 240
12.2.3 Pseudo Empirical Likelihood 242
12.2.4 Reverse Information 244
12.2.5 The Truncated Linear Method 245
12.2.6 General Pseudo-Distance 246
12.2.7 The Logistic Method 249
12.2.8 Deville Calibration Function 249
12.2.9 Roy and Vanheuverzwyn Method 251
12.3 Solving Calibration Equations 252
12.3.1 Solving by Newton’s Method 252
12.3.2 Bound Management 253
12.3.3 Improper Calibration Functions 254
12.3.4 Existence of a Solution 254
12.4 Calibrating on Households and Individuals 255
12.5 Generalized Calibration 256
12.5.1 Calibration Equations 256
12.5.2 Linear Calibration Functions 257
12.6 Calibration in Practice 258
12.7 An Example 259
Exercises 260
13 Model-Based approach 263
13.1 Model Approach 263
13.2 The Model 263
13.3 Homoscedastic Constant Model 267
13.4 Heteroscedastic Model 1 Without Intercept 267
13.5 Heteroscedastic Model 2 Without Intercept 269
13.6 Univariate Homoscedastic Linear Model 270
13.7 Stratified Population 271
13.8 Simplified Versions of the Optimal Estimator 273
13.9 Completed Heteroscedasticity Model 276
13.10 Discussion 277
13.11 An Approach that is Both Model- and Design-based 277
14 Estimation of Complex Parameters 281
14.1 Estimation of a Function of Totals 281
14.2 Variance Estimation 282
14.3 Covariance Estimation 283
14.4 Implicit Function Estimation 283
14.5 Cumulative Distribution Function and Quantiles 284
14.5.1 Cumulative Distribution Function Estimation 284
14.5.2 Quantile Estimation: Method 1 284
14.5.3 Quantile Estimation: Method 2 285
14.5.4 Quantile Estimation: Method 3 287
14.5.5 Quantile Estimation: Method 4 288
14.6 Cumulative Income, Lorenz Curve, and Quintile Share Ratio 288
14.6.1 Cumulative Income Estimation 288
14.6.2 Lorenz Curve Estimation 289
14.6.3 Quintile Share Ratio Estimation 289
14.7 Gini Index 290
14.8 An Example 291
15 Variance Estimation by Linearization 295
15.1 Introduction 295
15.2 Orders of Magnitude in Probability 295
15.3 Asymptotic Hypotheses 300
15.3.1 Linearizing a Function of Totals 301
15.3.2 Variance Estimation 303
15.4 Linearization of Functions of Interest 303
15.4.1 Linearization of a Ratio 303
15.4.2 Linearization of a Ratio Estimator 304
15.4.3 Linearization of a Geometric Mean 305
15.4.4 Linearization of a Variance 305
15.4.5 Linearization of a Covariance 306
15.4.6 Linearization of a Vector of Regression Coefficients 307
15.5 Linearization by Steps 308
15.5.1 Decomposition of Linearization by Steps 308
15.5.2 Linearization of a Regression Coefficient 308
15.5.3 Linearization of a Univariate Regression Estimator 309
15.5.4 Linearization of a Multiple Regression Estimator 309
15.6 Linearization of an Implicit Function of Interest 310
15.6.1 Estimating Equation and Implicit Function of Interest 310
15.6.2 Linearization of a Logistic Regression Coefficient 311
15.6.3 Linearization of a Calibration Equation Parameter 313
15.6.4 Linearization of a Calibrated Estimator 313
15.7 Influence Function Approach 314
15.7.1 Function of Interest, Functional 314
15.7.2 Definition 315
15.7.3 Linearization of a Total 316
15.7.4 Linearization of a Function of Totals 316
15.7.5 Linearization of Sums and Products 317
15.7.6 Linearization by Steps 318
15.7.7 Linearization of a Parameter Defined by an Implicit Function 318
15.7.8 Linearization of a Double Sum 319
15.8 Binder’s Cookbook Approach 321
15.9 Demnati and Rao Approach 322
15.10 Linearization by the Sample Indicator Variables 324
15.10.1 The Method 324
15.10.2 Linearization of a Quantile 326
15.10.3 Linearization of a Calibrated Estimator 327
15.10.4 Linearization of a Multiple Regression Estimator 328
15.10.5 Linearization of an Estimator of a Complex Function with Calibrated Weights 329
15.10.6 Linearization of the Gini Index 330
15.11 Discussion on Variance Estimation 331
Exercises 331
16 Treatment of Nonresponse 333
16.1 Sources of Error 333
16.2 Coverage Errors 334
16.3 Different Types of Nonresponse 334
16.4 Nonresponse Modeling 335
16.5 Treating Nonresponse by Reweighting 336
16.5.1 Nonresponse Coming from a Sample 336
16.5.2 Modeling the Nonresponse Mechanism 337
16.5.3 Direct Calibration of Nonresponse 339
16.5.4 Reweighting by Generalized Calibration 341
16.6 Imputation 342
16.6.1 General Principles 342
16.6.2 Imputing From an Existing Value 342
16.6.3 Imputation by Prediction 342
16.6.4 Link Between Regression Imputation and Reweighting 343
16.6.5 Random Imputation 345
16.7 Variance Estimation with Nonresponse 347
16.7.1 General Principles 347
16.7.2 Estimation by Direct Calibration 348
16.7.3 General Case 349
16.7.4 Variance for Maximum Likelihood Estimation 350
16.7.5 Variance for Estimation by Calibration 353
16.7.6 Variance of an Estimator Imputed by Regression 356
16.7.7 Other Variance Estimation Techniques 357
17 Summary Solutions to the Exercises 359
Bibliography 379
Author Index 405
Subject Index 411
YVES TILLÉ, PhD, is a Professor at the University of Neuchâtel (Université de Neuchâtel) in Neuchâtel, Switzerland.
Date de parution : 03-2020
Ouvrage de 448 p.
17.5x24.6 cm