The Basics of Financial Econometrics Tools, Concepts, and Asset Management Applications Frank J. Fabozzi Series
Auteurs : Fabozzi Frank J., Focardi Sergio M., Rachev Svetlozar T., Arshanapalli Bala G.
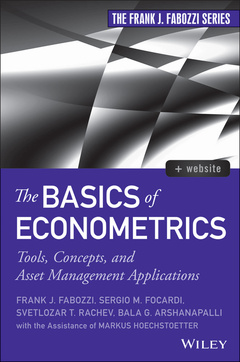
As finance and financial products have become more complex, financial econometrics has emerged as a fast-growing field and necessary foundation for anyone involved in quantitative finance. The techniques of financial econometrics facilitate the development and management of new financial instruments by providing models for pricing and risk assessment. In short, financial econometrics is an indispensable component to modern finance.
The Basics of Financial Econometrics covers the commonly used techniques in the field without using unnecessary mathematical/statistical analysis. It focuses on foundational ideas and how they are applied. Topics covered include: regression models, factor analysis, volatility estimations, and time series techniques.
- Covers the basics of financial econometrics?an important topic in quantitative finance
- Contains several chapters on topics typically not covered even in basic books on econometrics such as model selection, model risk, and mitigating model risk
Geared towards both practitioners and finance students who need to understand this dynamic discipline, but may not have advanced mathematical training, this book is a valuable resource on a topic of growing importance.
Acknowledgments xvii
About the Authors xix
Chapter 1 Introduction 1
Financial Econometrics at Work 2
The Data Generating Process 5
Applications of Financial Econometrics to Investment Management 6
Key Points 10
Chapter 2 Simple Linear Regression 13
The Role of Correlation 13
Regression Model: Linear Functional Relationship between Two Variables 14
Distributional Assumptions of the Regression Model 16
Estimating the Regression Model 18
Goodness-of-Fit of the Model 22
Two Applications in Finance 25
Linear Regression of a Nonlinear Relationship 36
Key Points 38
CHAPTER 3 Multiple Linear Regression 41
The Multiple Linear Regression Model 42
Assumptions of the Multiple Linear Regression Model 43
Estimation of the Model Parameters 43
Designing the Model 45
Diagnostic Check and Model Significance 46
Applications to Finance 51
Key Points 79
chapter 4 Building and Testing a Multiple Linear Regression Model 81
The Problem of Multicollinearity 81
Model Building Techniques 84
Testing the Assumptions of the Multiple Linear Regression Model 88
Key Points 100
CHAPTER 5 Introduction to Time Series Analysis 103
What Is a Time Series? 103
Decomposition of Time Series 104
Representation of Time Series with Difference Equations 108
Application: The Price Process 109
Key Points 113
chapter 6 Regression Models with Categorical Variables 115
Independent Categorical Variables 116
Dependent Categorical Variables 137
Key Points 140
Chapter 7 Quantile Regressions 143
Limitations of Classical Regression Analysis 144
Parameter Estimation 144
Quantile Regression Process 146
Applications of Quantile Regressions in Finance 148
Key Points 155
CHAPTER 8 Robust Regressions 157
Robust Estimators of Regressions 158
Illustration: Robustness of the
Corporate Bond Yield Spread Model 161
Robust Estimation of Covariance and Correlation Matrices 166
Applications 168
Key Points 170
Chapter 9 Autoregressive Moving Average Models 171
Autoregressive Models 172
Moving Average Models 176
Autoregressive Moving Average Models 178
ARMA Modeling to Forecast S&P 500 Weekly Index Returns 181
Vector Autoregressive Models 188
Key Points 189
Chapter 10 Cointegration 191
Stationary and Nonstationary Variables and Cointegration 192
Testing for Cointegration 196
Key Points 211
chapter 11 Autoregressive Heteroscedasticity Model and Its Variants 213
Estimating and Forecasting Volatility 214
ARCH Behavior 215
GARCH Model 223
What Do ARCH/GARCH Models Represent? 226
Univariate Extensions of GARCH Modeling 226
Estimates of ARCH/GARCH Models 229
Application of GARCH Models to Option Pricing 230
Multivariate Extensions of ARCH/GARCH Modeling 231
Key Points 233
Chapter 12 Factor Analysis and Principal Components Analysis 235
Assumptions of Linear Regression 236
Basic Concepts of Factor Models 237
Assumptions and Categorization of Factor Models 240
Similarities and Differences between Factor Models and Linear Regression 241
Properties of Factor Models 242
Estimation of Factor Models 244
Principal Components Analysis 251
Differences between Factor Analysis and PCA 259
Approximate (Large) Factor Models 261
Approximate Factor Models and PCA 263
Key Points 264
Chapter 13 Model Estimation 265
Statistical Estimation and Testing 265
Estimation Methods 267
Least-Squares Estimation Method 268
The Maximum Likelihood Estimation Method 278
Instrumental Variables 283
Method of Moments 284
The M-Estimation Method and M-Estimators 289
Key Points 289
CHAPTER 14 Model Selection 291
Physics and Economics: Two Ways of Making Science 291
Model Complexity and Sample Size 293
Data Snooping 296
Survivorship Biases and Other Sample Defects 297
Model Risk 300
Model Selection in a Nutshell 301
Key Points 303
Chapter 15 Formulating and Implementing Investment Strategies Using Financial Econometrics 305
The Quantitative Research Process 307
Investment Strategy Process 314
Key Points 318
Appendix A Descriptive Statistics 321
Basic Data Analysis 321
Measures of Location and Spread 328
Multivariate Variables and Distributions 332
Appendix B Continuous Probability Distributions Commonly Used in Financial Econometrics 343
Normal Distribution 344
Chi-Square Distribution 347
Student’s t-Distribution 349
F-Distribution 352
α-Stable Distribution 353
Appendix C Inferential Statistics 359
Point Estimators 359
Confidence Intervals 369
Hypothesis Testing 372
Appendix D Fundamentals of Matrix Algebra 385
Vectors and Matrices Defined 385
Square Matrices 387
Determinants 388
Systems of Linear Equations 389
Linear Independence and Rank 391
Vector and Matrix Operations 391
Eigenvalues and Eigenvectors 396
APPENDIX E Model Selection Criterion: AIC and BIC 399
Akaike Information Criterion 400
Bayesian Information Criterion 402
Appendix F Robust Statistics 405
Robust Statistics Defined 405
Qualitative and Quantitative Robustness 406
Resistant Estimators 406
M-Estimators 408
The Least Median of Squares Estimator 408
The Least Trimmed of Squares Estimator 409
Robust Estimators of the Center 409
Robust Estimators of the Spread 410
Illustration of Robust Statistics 410
Index 413
FRANK J. FABOZZI is Professor of Finance at EDHEC Business School and Editor of the Journal of Portfolio Management.
SERGIO M. FOCARDI is Visiting Professor of Finance at Stony Brook University and a founding partner of the Paris-based consulting firm The Intertek Group.
SVETLOZAR T. RACHEV is Professor of Finance, College of Business and Center for Finance, Stony Brook University, and Chief-Scientist with FinAnalytica.
BALA G. ARSHANAPALLI is the Gallagher-Mills Chair of Business and Economics at Indiana University Northwest.
Date de parution : 03-2014
Ouvrage de 448 p.
16x23.6 cm
Thème de The Basics of Financial Econometrics :
Mots-clés :
frank fabozzi; fabozzi series; sergio focardi; svetlozar rachev; bala arshanapalli; basics of econometrics; econometrics basics; financial econometric basics; financial econometrics basics; probability theory; linear dependency measures; simple linear regression; time series; multivariate linear regression model; regression topics; quantile regressions; logistic regressions; factor analysis; principal component analysis; estimating volatility; time series techniques; model selection; model risk