Prognostics and Remaining Useful Life (RUL) Estimation Predicting with Confidence
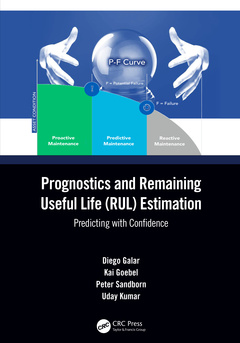
Maintenance combines various methods, tools, and techniques in a bid to reduce maintenance costs while increasing the reliability, availability, and security of equipment. Condition-based maintenance (CBM) is one such method, and prognostics forms a key element of a CBM program based on mathematical models for predicting remaining useful life (RUL). Prognostics and Remaining Useful Life (RUL) Estimation: Predicting with Confidence compares the techniques and models used to estimate the RUL of different assets, including a review of the relevant literature on prognostic techniques and their use in the industrial field. This book describes different approaches and prognosis methods for different assets backed up by appropriate case studies.
FEATURES
- Presents a compendium of RUL estimation methods and technologies used in predictive maintenance
- Describes different approaches and prognosis methods for different assets
- Includes a comprehensive compilation of methods from model-based and data-driven to hybrid
- Discusses the benchmarking of RUL estimation methods according to accuracy and uncertainty, depending on the target application, the type of asset, and the forecast performance expected
- Contains a toolset of methods and a way of deployment aimed at a versatile audience
This book is aimed at professionals, senior undergraduates, and graduate students in all interdisciplinary engineering streams that focus on prognosis and maintenance.
1. Information in Maintenance 2. Predictive Maintenance Programs and Servitization Maintenance as a Service (MaaS) Creating Value through Prognosis Capabilities 3. RUL Estimation Powered by Data-Driven Techniques 4. Context Awareness and Situation Awareness in Prognostics 5. Black Swans and Physics of Failure 6. Hybrid Prognostics Combining Physics-Based and Data-Driven Approaches 7. Prognosis in Prescriptive Analytics 8. Uncertainty Management and the Confidence of RUL Predictions 9. RUL Estimation of Dynamic and Static Assets 10. Principles of Digital Twin 11. Application of Prognosis in Industry, Energy, and Transportation
Dr. Diego Galar is Full Professor of Condition Monitoring in the Division of Operation and Maintenance Engineering at LTU, Luleå University of Technology where he is coordinating several H2020 projects related to different aspects of cyber physical systems, Industry 4.0, IoT or Industrial AI and Big Data. He was also involved in the SKF UTC centre located in Lulea focused on SMART bearings and also actively involved in national projects with the Swedish industry or funded by Swedish national agencies like Vinnova.
He is also principal researcher in Tecnalia (Spain), heading the Maintenance and Reliability research group within the Division of Industry and Transport.
He has authored more than five hundred journal and conference papers, books and technical reports in the field of maintenance, working also as member of editorial boards, scientific committees and chairing international journals and conferences and actively participating in national and international committees for standardization and R&D in the topics of reliability and maintenance.
In the international arena, he has been visiting Professor in the Polytechnic of Braganza (Portugal), University of Valencia and NIU (USA) and the Universidad Pontificia Católica de Chile. Currently, he is visiting professor in University of Sunderland (UK), University of Maryland (USA), and Chongqing University in China.
Dr. Kai Goebel is a Principal Scientist in the System Sciences Lab at Palo Alto Research Center (PARC). His interest is broadly in condition-based maintenance and systems health management for a broad spectrum of cyber-physical systems in the transportation, energy, aerospace, defense, and manufacturing sectors. Prior to joining PARC, Dr. Goebel worked at NASA Ames Research Center and General Electric Corporate Research & Development center. At NASA, he was a branch chief leading the Discovery and Systems Health tech area which included groups f
Date de parution : 12-2021
17.8x25.4 cm
Thème de Prognostics and Remaining Useful Life (RUL) Estimation :
Mots-clés :
RUL Prediction; Condition Based Maintenance; RUL Estimation; Predictive Maintenance; Digital Twin; Industry 4.0; Kalman Filters; Data Analytics; Prescriptive Analytics; Epistemic Uncertainty; Complex Systems; Aleatory Uncertainty; Digital Twins; PdM; Anomaly Detection Algorithms; Business Processes; Ml Model; SCADA System; DBN; Physics Based Models; CPSs; RVM; BG Model; Context Aware Applications; Sensor Fusion; Uncertainty Quantification; Corrective Maintenance; Hybrid Prognostics Approach; Predictive Analytics; Mars; Work Order