Healthcare Analytics From Data to Knowledge to Healthcare Improvement Wiley Series in Operations Research and Management Science Series
Coordonnateurs : Yang Hui, Lee Eva K.
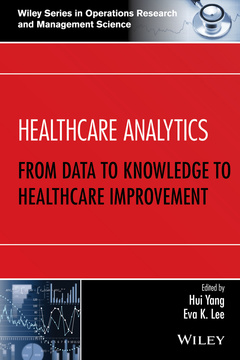
Features of statistical and operational research methods and tools being used to improve the healthcare industry
With a focus on cutting-edge approaches to the quickly growing field of healthcare, Healthcare Analytics: From Data to Knowledge to Healthcare Improvement provides an integrated and comprehensive treatment on recent research advancements in data-driven healthcare analytics in an effort to provide more personalized and smarter healthcare services. Emphasizing data and healthcare analytics from an operational management and statistical perspective, the book details how analytical methods and tools can be utilized to enhance healthcare quality and operational efficiency.
Organized into two main sections, Part I features biomedical and health informatics and specifically addresses the analytics of genomic and proteomic data; physiological signals from patient-monitoring systems; data uncertainty in clinical laboratory tests; predictive modeling; disease modeling for sepsis; and the design of cyber infrastructures for early prediction of epidemic events. Part II focuses on healthcare delivery systems, including system advances for transforming clinic workflow and patient care; macro analysis of patient flow distribution; intensive care units; primary care; demand and resource allocation; mathematical models for predicting patient readmission and postoperative outcome; physician?patient interactions; insurance claims; and the role of social media in healthcare. Healthcare Analytics: From Data to Knowledge to Healthcare Improvement also features:
? Contributions from well-known international experts who shed light on new approaches in this growing area
? Discussions on contemporary methods and techniques to address the handling of rich and large-scale healthcare data as well as the overall optimization of healthcare system operations
? Numerous real-world examples and case studies that emphasize the vast potential of statistical and operational research tools and techniques to address the big data environment within the healthcare industry
? Plentiful applications that showcase analytical methods and tools tailored for successful healthcare systems modeling and improvement
The book is an ideal reference for academics and practitioners in operations research, management science, applied mathematics, statistics, business, industrial and systems engineering, healthcare systems, and economics. Healthcare Analytics: From Data to Knowledge to Healthcare Improvement is also appropriate for graduate-level courses typically offered within operations research, industrial engineering, business, and public health departments.
List of Contributors xvii
Preface xxi
Part I Advances In Biomedical And Health Informatics 1
1 Recent Development in Methodology for Gene Network Problems and Inferences 3
Sung W. Han and Hua Zhong
1.1 Introduction 3
1.2 Background 5
1.3 Genetic Data Available 7
1.4 Methodology 7
1.4.1 Structural Equation Model 8
1.4.2 Score Function Formulation 9
1.4.3 Two-Stage Learning 12
1.4.4 Further Issues 13
1.5 Search Algorithm 13
1.5.1 Global Optimal Solution Search 13
1.5.2 Heuristic Algorithm for a Local Optimal Solution 14
1.6 PC Algorithm 15
1.7 Application/Case Studies 16
1.7.1 Skin Cutaneous Melanoma (SKCM) Data from the TCGA Data Portal Website 16
1.7.2 The CCLE (Cancer Cell Line Encyclopedia) Project 20
1.7.3 Cellular Signaling Network in Flow Cytometry Data 20
1.8 Discussion 23
1.9 Other Useful Softwares 23
Acknowledgments 24
References 24
2 Biomedical Analytics and Morphoproteomics: An Integrative Approach for Medical Decision Making for Recurrent or Refractory Cancers 31
Mary F. McGuire and Robert E. Brown
2.1 Introduction 31
2.2 Background 32
2.2.1 Data 33
2.2.2 Tools 33
2.2.3 Algorithms 34
2.2.4 Literature Review 35
2.3 Methodology 37
2.3.1 Morphoproteomics (Fig. 2.1(1–3)) 39
2.3.2 Biomedical Analytics (Fig. 2.1(4–10)) 40
2.3.3 Integrating Morphoproteomics and Biomedical Analytics 44
2.4 Case Studies 46
2.4.1 Clinical: Therapeutic Recommendations for Pancreatic Adenocarcinoma 46
2.4.2 Clinical: Biology Underlying Exceptional Responder in Refractory Hodgkin’s Lymphoma 48
2.4.3 Research: Role of the Hypoxia Pathway in Both Oncogenesis and Embryogenesis 50
2.5 Discussion 51
2.6 Conclusions 52
Acknowledgments 53
References 53
3 Characterization and Monitoring of Nonlinear Dynamics and Chaos in Complex Physiological Systems59
Hui Yang, Yun Chen, and Fabio Leonelli
3.1 Introduction 59
3.2 Background 61
3.3 Sensor-Based Characterization and Modeling of Nonlinear Dynamics 65
3.3.1 Multifractal Spectrum Analysis of Nonlinear Time Series 65
3.3.2 Recurrence Quantification Analysis 75
3.3.3 Multiscale Recurrence Quantification Analysis 78
3.4 Healthcare Applications 80
3.4.1 Nonlinear Characterization of Heart Rate Variability 81
3.4.2 Multiscale Recurrence Analysis of Space–Time Physiological Signals 85
3.5 Summary 88
Acknowledgments 90
References 90
4 Statistical Modeling of Electrocardiography Signal for Subject Monitoring and Diagnosis 95
Lili Chen, Changyue Song, and Xi Zhang
4.1 Introduction 95
4.2 Basic Elements of ECG 96
4.3 Statistical Modeling of ECG for Disease Diagnosis 99
4.3.1 ECG Signal Denoising 100
4.3.2 Waveform Detection 105
4.3.3 Feature Extraction 106
4.3.4 Disease Classification and Diagnosis 111
4.4 An Example: Detection of Obstructive Sleep Apnea from a Single ECG Lead 115
4.4.1 Introduction to Obstructive Sleep Apnea 115
4.5 Materials and Methods 115
4.5.1 Database 115
4.5.2 QRS Detection and RR Correction 116
4.5.3 R Wave Amplitudes and EDR Signal 117
4.5.4 Feature Set 117
4.5.5 Classifier Training with Feature Selection 118
4.6 Results 118
4.6.1 QRS Detection and RR Correction 118
4.6.2 Feature Selection 118
4.6.3 OSA Detection 120
4.7 Conclusions and Discussions 121
References 121
5 Modeling and Simulation of Measurement Uncertainty in Clinical Laboratories 127
Varun Ramamohan, James T. Abbott, and Yuehwern Yih
5.1 Introduction 127
5.2 Background and Literature Review 129
5.2.1 Measurement Uncertainty: Background and Analytical Estimation 130
5.2.2 Uncertainty in Clinical Laboratories 134
5.2.3 Uncertainty in Clinical Laboratories: A System Approach 136
5.3 Model Development Guidelines 138
5.3.1 System Description and Process Phases 138
5.3.2 Modeling Guidelines 139
5.4 Implementation of Guidelines: Enzyme Assay Uncertainty Model 141
5.4.1 Calibration Phase 142
5.4.2 Sample Analysis Phase 149
5.4.3 Results and Analysis 150
5.5 Discussion and Conclusions 152
References 154
6 Predictive Analytics: Classification in Medicine and Biology 159
Eva K. Lee
6.1 Introduction 159
6.2 Background 161
6.3 Machine Learning with Discrete Support Vector Machine Predictive Models 163
6.3.1 Modeling of Reserved-Judgment Region for General Groups 164
6.3.2 Discriminant Analysis via Mixed-Integer Programming 165
6.3.3 Model Variations 167
6.3.4 Theoretical Properties and Computational Strategies 170
6.4 Applying DAMIP to Real-World Applications 170
6.4.1 Validation of Model and Computational Effort 171
6.4.2 Applications to Biological and Medical Problems 171
6.5 Summary and Conclusion 182
Acknowledgments 183
References 183
7 Predictive Modeling in Radiation Oncology 189
Hao Zhang, Robert Meyer, Leyuan Shi, Wei Lu, and Warren D’Souza
7.1 Introduction 189
7.2 Tutorials of Predictive Modeling Techniques 191
7.2.1 Feature Selection 191
7.2.2 Support Vector Machine 192
7.2.3 Logistic Regression 193
7.2.4 Decision Tree 193
7.3 Review of Recent Predictive Modeling Applications in Radiation Oncology 194
7.3.1 Machine Learning for Medical Image Processing 194
7.3.2 Machine Learning in Real-Time Tumor Localization 196
7.3.3 Machine Learning for Predicting Radiotherapy Response 197
7.4 Modeling Pathologic Response of Esophageal Cancer to Chemoradiotherapy 199
7.4.1 Input Features 200
7.4.2 Feature Selection and Predictive Model Construction 200
7.4.3 Results 202
7.4.4 Discussion 204
7.5 Modeling Clinical Complications after Radiation Therapy 205
7.5.1 Dose-Volume Thresholds: Relationship to OAR Complications 205
7.5.2 Modeling the Radiation-Induced Complications via Treatment Plan Surface 206
7.5.3 Modeling Results 208
7.6 Modeling Tumor Motion with Respiratory Surrogates 211
7.6.1 Cyberknife System Data 211
7.6.2 Modeling for the Prediction of Tumor Positions 212
7.6.3 Results of Tumor Positions Modeling 212
7.6.4 Discussion 214
7.7 Conclusion 215
References 215
8 Mathematical Modeling of Innate Immunity Responses of Sepsis: Modeling and Computational Studies 221
Chih-Hang J. Wu, Zhenzhen Shi, David Ben-Arieh, and Steven Q. Simpson
8.1 Background 221
8.2 System Dynamic Mathematical Model (SDMM) 223
8.3 Pathogen Strain Selection 224
8.3.1 Step 1: Kupffer Local Response Model 224
8.3.2 Step 2: Neutrophils Immune Response Model 228
8.3.3 Step 3: Damaged Tissue Model 233
8.3.4 Step 4: Monocytes Immune Response Model 234
8.3.5 Step 5: Anti-inflammatory Immune Response Model 237
8.4 Mathematical Models of Innate Immunity of AIR 239
8.4.1 Inhibition of Anti-inflammatory Cytokines 239
8.4.2 Mathematical Model of Innate Immunity of AIR 239
8.4.3 Stability Analysis 241
8.5 Discussion 247
8.5.1 Effects of Initial Pathogen Load on Sepsis Progression 247
8.5.2 Effects of Pro- and Anti-inflammatory Cytokines on Sepsis Progression 250
8.6 Conclusion 254
References 254
Part II Analytics for Healthcare Delivery 261
9 Systems Analytics: Modeling and Optimizing ClinicWorkflow and Patient Care 263
Eva K. Lee, Hany Y. Atallah, Michael D. Wright, Calvin Thomas IV, Eleanor T. Post, Daniel T. Wu, and Leon L. Haley Jr
9.1 Introduction 264
9.2 Background 266
9.3 Challenges and Objectives 267
9.4 Methods and Design of Study 268
9.4.1 ED Workflow and Services 269
9.4.2 Data Collection and Time-Motion Studies 270
9.4.3 Machine Learning for Predicting Patient Characteristics and Return Patterns 274
9.4.4 The Computerized ED System Workflow Model 277
9.4.5 Model Validation 282
9.5 Computational Results, Implementation, and ED Performance Comparison 285
9.5.1 Phase I: Results 285
9.5.2 Phase I: Adoption and Implementation 288
9.5.3 Phase II: Results 288
9.5.4 Phase II: Adoption and Implementation 290
9.6 Benefits and Impacts 292
9.6.1 Quantitative Benefits 294
9.6.2 Qualitative Benefits 296
9.7 Scientific Advances 297
9.7.1 Hospital Care Delivery Advances 297
9.7.2 OR Advances 298
Acknowledgments 298
References 299
10 A Multiobjective Simulation Optimization of the Macrolevel Patient Flow Distribution 303
Yunzhe Qiu and Jie Song
10.1 Introduction 303
10.2 Literature Review 305
10.2.1 Simulation Modeling on Patient Flow 305
10.2.2 Multiobjective Patient Flow Optimization Problems 306
10.2.3 Simulation Optimization 307
10.3 Problem Description and Modeling 308
10.3.1 Problem Description 308
10.3.2 System Modeling 310
10.4 Methodology 312
10.4.1 Simulation Model Description 312
10.4.2 Optimization 313
10.5 Case Study: Adjusting Patient Flow for a Two-Level Healthcare System Centered on the Puth 316
10.5.1 Background and Data 316
10.5.2 Simulation under Current Situation 318
10.5.3 Model Validation 320
10.5.4 Optimization through Algorithm 1 321
10.5.5 Optimization through Algorithm 2 322
10.5.6 Comparison of the Two Algorithms 327
10.5.7 Managerial Insights and Recommendations 328
10.6 Conclusions and the Future Work 329
Acknowledgments 330
References 331
11 Analysis of Resource Intensive Activity Volumes in US Hospitals 335
Shivon Boodhoo and Sanchoy Das
11.1 Introduction 335
11.2 Structural Classification of Hospitals 337
11.3 Productivity Analysis of Hospitals 339
11.4 Resource and Activity Database for US Hospitals 341
11.4.1 Medicare Data Sources for Hospital Operations 343
11.5 Activity-Based Modeling of Hospital Operations 344
11.5.1 Direct Care Activities 344
11.5.2 The Hospital Unit of Care (HUC) Model 347
11.5.3 HUC Component Results by State 350
11.6 Resource use Profile of Hospitals from HUC Activity Data 351
11.6.1 Comparing the Resource Use Profile of States 353
11.6.2 Application of the Hospital Classification Rules 355
11.7 Summary 357
References 358
12 Discrete-Event Simulation for Primary Care Redesign: Review and a Case Study 361
Xiang Zhong, Molly Williams, Jingshan Li, Sally A. Kraft, and Jeffrey S. Sleeth
12.1 Introduction 361
12.2 Review of Relevant Literature 362
12.2.1 Literature on Primary Care Redesign 362
12.2.2 Literature on Discrete-Event Simulation in Healthcare 366
12.2.3 UW Health Improvement Projects 369
12.3 A Simulation Case Study at a Pediatric Clinic 369
12.3.1 Patient Flow 369
12.3.2 Model Development 371
12.3.3 Model Validation 376
12.4 What–If Analyses 376
12.4.1 Staffing Analysis 376
12.4.2 Resident Doctor 377
12.4.3 Schedule Template Change 377
12.4.4 Volume Change 379
12.4.5 Room Assignment 379
12.4.6 Early Start 380
12.4.7 Additional Observations 382
12.5 Conclusions 382
References 382
13 Temporal and Spatiotemporal Models for Ambulance Demand 389
Zhengyi Zhou and David S. Matteson
13.1 Introduction 389
13.2 Temporal Ambulance Demand Estimation 391
13.2.1 Notation 392
13.2.2 Factor Modeling with Constraints and Smoothing 393
13.2.3 Adaptive Forecasting with Time Series Models 395
13.3 Spatiotemporal Ambulance Demand Estimation 398
13.3.1 Spatiotemporal Finite Mixture Modeling 400
13.3.2 Estimating Ambulance Demand 403
13.3.3 Model Performance 405
13.4 Conclusions 409
References 410
14 Mathematical Optimization and Simulation Analyses for Optimal Liver Allocation Boundaries 413
Naoru Koizumi, Monica Gentili, Rajesh Ganesan, Debasree DasGupta, Amit Patel, Chun-Hung Chen, Nigel Waters, and Keith Melancon
14.1 Introduction 414
14.2 Methods 416
14.2.1 Mathematical Model: Optimal Locations of Transplant Centers and OPO Boundaries 416
14.2.2 Discrete-Event Simulation: Evaluation of Optimal OPO Boundaries 422
14.3 Results 423
14.3.1 New Locations of Transplant Centers 423
14.3.2 New OPO Boundaries 426
14.3.3 Evaluation of New OPO Boundaries 428
14.4 Conclusions 433
Acknowledgment 435
References 435
15 Predictive Analytics in 30-Day Hospital Readmissions for Heart Failure Patients 439
Si-Chi Chin, Rui Liu, and Senjuti B. Roy
15.1 Introduction 440
15.2 Analytics in Prediction Hospital Readmission Risk 441
15.2.1 The Overall Prediction Pipeline 441
15.2.2 Data Preprocessing 441
15.2.3 Predictive Models 442
15.2.4 Experiment and Evaluation 444
15.3 Analytics in Recommending Intervention Strategies 447
15.3.1 The Overall Intervention Pipeline 447
15.3.2 Bayesian Network Construction 448
15.3.3 Recommendation Rule Generation 452
15.3.4 Intervention Recommendation 453
15.3.5 Experiments 454
15.4 Related Work 457
15.5 Conclusion 459
References 459
16 Heterogeneous Sensing and Predictive Modeling of Postoperative Outcomes 463
Yun Chen, Fabio Leonelli, and Hui Yang
16.1 Introduction 463
16.2 Research Background 466
16.2.1 Acute Physiology and Chronic Health Evaluation (APACHE) 466
16.2.2 Simplified Acute Physiology Score (SAPS) 469
16.2.3 Mortality Probability Model (MPM) 470
16.2.4 Sequential Organ Failure Assessment (SOFA) 472
16.3 Research Methodology 474
16.3.1 Data Categorization 475
16.3.2 Data Preprocessing and Missing Data Imputation 475
16.3.3 Feature Extraction 482
16.3.4 Feature Selection 484
16.3.5 Predictive Model 487
16.3.6 Cross-Validation and Ensemble Voting Processes 489
16.4 Materials and Experimental Design 491
16.5 Experimental Results 491
16.6 Discussion and Conclusions 498
Acknowledgments 499
References 499
17 Analyzing Patient–Physician Interaction in Consultation for Shared Decision Making 503
Thembi Mdluli, Joyatee Sarker, Carolina Vivas-Valencia, Nan Kong, and Cleveland G. Shields
17.1 Introduction 503
17.2 Literature Review 505
17.2.1 Patient–Physician Interaction on Prognosis Discussion 506
17.2.2 Physician–Patient Interaction on Pain Assessment 509
17.3 Our Recent Data Mining Studies 510
17.3.1 Predicting Patient Satisfaction with Survey Data 510
17.3.2 Predicting Patient Satisfaction with Conservation Data 513
17.4 Future Directions 515
17.4.1 Regression Shrinkage and Selection 515
17.4.2 Conversational Characterization 517
17.5 Concluding Remarks 519
References 520
18 The History and Modern Applications of Insurance Claims Data in Healthcare Research 523
Margrét V. Bjarndóttir, David Czerwinski, and Yihan Guan
18.1 Introduction 523
18.1.1 Advantages and Limitations of Claims Data 525
18.1.2 Application Areas 526
18.1.3 Statistical Methodologies Used in Claims-Based Studies 528
18.2 Healthcare Cost Predictions 531
18.2.1 Modeling of Healthcare Costs 531
18.2.2 Modeling of Disease Burden and Interactions 533
18.2.3 Performance Measures and Baselines 534
18.2.4 Prediction Algorithms 534
18.2.5 Applying Regression Trees to Cost Predictions 535
18.2.6 Applying Clustering Algorithms to Cost Predictions 537
18.2.7 Identifying High-Cost Members 539
18.2.8 Discussion 539
18.3 Measuring Quality of Care 540
18.3.1 Structure, Process, and Outcomes 540
18.3.2 The Quality of Quality Data 542
18.3.3 Composite Quality Measures 542
18.3.4 Practical Considerations for Constructing Quality Scores 544
18.3.5 A Statistical Approach to Measuring Quality 545
18.3.6 Quality as a Case Management Tool 546
18.3.7 Discussion 547
18.4 Conclusions 548
References 548
19 Understanding the Role of Social Media in Healthcare via Analytics: a Health Plan Perspective 555
Sinjini Mitra and Rema Padman
19.1 Introduction 555
19.2 Literature Review 556
19.2.1 Privacy and Security Concerns in Social Media and Healthcare 559
19.2.2 Analytics in Healthcare and Social Media 561
19.3 Case Study Description 562
19.3.1 Survey Design 563
19.4 Research Methods and Analytics Tools 564
19.4.1 The Logistic Regression Model 564
19.5 Results and Discussions 568
19.5.1 Descriptive Statistics 568
19.5.2 Baseline of Technology Usage 570
19.5.3 Mobile and Social Media Usage 571
19.5.4 Clustering of Member Population by Technology, Social, and Mobile Media Usage 572
19.5.5 Interest in Adopting Online Tools for Healthcare Purposes 573
19.5.6 Interest in Adopting Mobile Apps for Healthcare Purposes 574
19.5.7 Health and Wellness Objectives 577
19.5.8 Privacy and Security Concerns 580
19.5.9 Predictive Models 581
19.6 Conclusions 584
References 585
Index 589
HUI YANG, PhD, is Associate Professor in the Harold and Inge Marcus Department of Industrial and Manufacturing Engineering at The Pennsylvania State University. His research interests include sensor-based modeling and analysis of complex systems for process monitoring/control; system diagnostics/ prognostics; quality improvement; and performance optimization with special focus on nonlinear stochastic dynamics and the resulting chaotic, recurrence, self-organizing behaviors.
EVA K. LEE, PhD, is Professor in the H. Milton Stewart School of Industrial and Systems Engineering at the Georgia Institute of Technology, Director of the Center for Operations Research in Medicine and HealthCare, and Distinguished Scholar in Health System, Health Systems Institute at both Emory University School of Medicine and Georgia Institute of Technology. Her research interests include health-risk prediction; early disease prediction and diagnosis; optimal treatment strategies and drug delivery; healthcare outcome analysis and treatment prediction; public health and medical preparedness; large-scale healthcare/medical decision analysis and quality improvement; clinical translational science; and business intelligence and organization transformation.
Date de parution : 08-2016
Ouvrage de 632 p.
16.3x24.1 cm
Thèmes de Healthcare Analytics :
Mots-clés :
healthcare; healthcare analytics; big data analytics; healthcare improvement; information technology; biostatistics; nonlinear dynamics; biomedical simulation and modeling; predictive modeling; healthcare systems simulation; simulation optimization; personalized healthcare; healthcare logistics; patient scheduling; healthcare organizational management and market analysis; chronic disease screening policy; treatment optimization; real-time patient monitoring; diagnostics; and prognostics; healthcare quality assessment and improvement; data mining; statistical learning methods; patient readmission